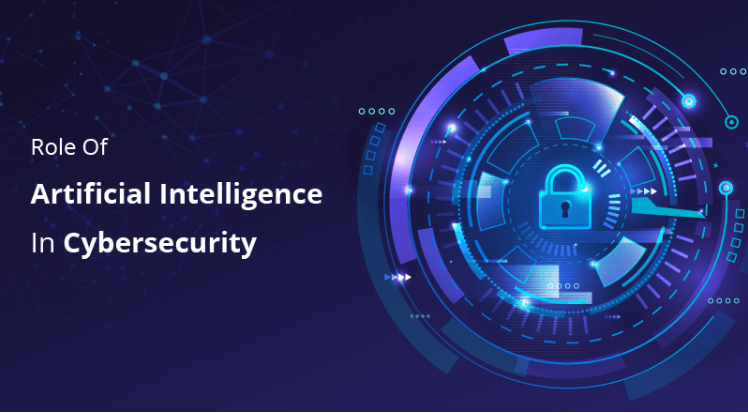
Role of Generative AI in Cybersecurity
- By ThreatMatrix
Introduction
Generative AI is reshaping the cybersecurity landscape in ways that few other technologies have. As cybersecurity threats grow more complex, businesses and security experts are increasingly relying on AI-driven tools to bolster defenses. The role of generative AI in cyber security, in particular, is emerging as a crucial tool that enhances cybersecurity protocols, improves threat detection, and automates response mechanisms. In this article, we’ll explore the top ways generative AI is playing a transformative role in cybersecurity.
1. Automating Threat Detection and Response
One of the most vital roles of generative AI in cybersecurity is its ability to automate threat detection and incident response. Traditional cybersecurity tools often rely on static rules or pre-defined behaviors, which can struggle to identify more sophisticated or evolving threats. Generative AI, with its deep learning capabilities, can analyze vast data sets in real-time, detect unusual patterns, and immediately flag potential security breaches.
Key Benefits:
- Real-time threat detection reduces response times.
- Automated analysis helps pinpoint anomalies and suspicious activity.
- By monitoring behavior patterns, generative AI tools can distinguish between normal user activity and potential threats, enhancing cybersecurity posture.
2. Enhancing Cybersecurity with Generative
Generative AI significantly enhances threat intelligence by synthesizing complex data patterns and predicting potential vulnerabilities. This predictive capability enables security teams to be proactive, rather than reactive, by identifying and addressing vulnerabilities before they are exploited.
How It Works:
- Generative AI models analyze global cyber threat data to identify patterns and emerging risks.
- Predictive insights generated by AI help cybersecurity teams develop strategies to defend against new attack vectors.
- As a result, organizations are better prepared to face ever-evolving cybersecurity threats.
3. Advanced Phishing Detection
Phishing attacks have become more sophisticated with cybercriminals increasingly using AI to generate authentic-looking emails and messages. Generative AI plays a critical role in defending against these attacks by recognizing patterns common in phishing content and flagging suspicious emails before they reach users.
Example:
- Generative AI models can analyze the context, tone, and language in emails to detect inconsistencies.
- By comparing emails to known phishing templates, AI can identify potential threats.
- Through natural language processing (NLP), generative AI also identifies unusual language that may indicate a phishing attempt.
4. Security in Deepfake Detection
The rise of deepfake technology has created significant challenges for cybersecurity, particularly in social engineering attacks. Generative AI helps mitigate these risks by developing algorithms that detect synthetic media. With the ability to analyze voice, image, and video patterns, AI can discern deepfakes from legitimate content, thereby safeguarding users and businesses from malicious impersonation attempts.
Impact on Cybersecurity:
- Generative AI uses data from real and synthetic sources to learn and identify signs of deepfakes.
- By integrating deepfake detection, cybersecurity systems can protect against social engineering attacks that use manipulated video or audio.
- This capability is crucial for protecting high-stakes sectors, such as finance and government, where deepfake attacks pose serious risks.
5. Adaptive Malware Defense
Traditional antivirus software often struggles with polymorphic malware that changes its code to evade detection. Generative AI can adapt to these threats by identifying and countering malware variants as they evolve. Using AI models trained on diverse malware samples, generative AI enables real-time defenses against complex malware.
Adaptive Approach:
- Generative AI detects patterns within malware code structures that indicate malicious intent, even if the code changes.
- AI-driven security solutions continuously learn from new threats, allowing for more effective defense against polymorphic attacks.
- This adaptability makes generative AI essential for malware detection, especially in sectors that handle sensitive data.
6. Supporting Zero Trust Security Models
Generative AI also enhances zero trust security models by continually analyzing user behaviors and establishing trust scores. In a zero-trust framework, every attempt to access resources is verified, even if the user is already within the network. Generative AI provides continuous authentication, detecting any deviation from standard behavior and issuing alerts for unauthorized access.
Benefits:
- AI models detect anomalies based on an individual’s typical behavior.
- Zero trust models supported by AI allow for more granular access control and fewer vulnerabilities.
- These capabilities are crucial in protecting against insider threats and unauthorized access attempts.
Conclusion
Generative AI has proven itself as an invaluable tool in cybersecurity, addressing challenges that traditional methods struggle to combat. From threat detection to deepfake identification, AI-driven technologies are improving cybersecurity protocols across industries. As generative AI continues to evolve, its role in cybersecurity will likely grow, providing ever-stronger defenses against the latest threats.
Embracing generative AI for cybersecurity is no longer optional; it’s essential. By staying informed about the latest advancements in AI and integrating these tools into security strategies, organizations can protect themselves from the increasingly sophisticated landscape of cyber threats in 2024.
Dark Web Monitoring for Healthcare
November 8, 2024[…] Role of Generative AI in Cybersecurity […]
Understanding Nation-State Cyber Threats
November 15, 2024[…] Technologies: Artificial intelligence and machine learning empower attackers to conduct more effective […]
Top Cybersecurity Trends in 2024: What Businesses Need to Know
January 20, 2025[…] Artificial Intelligence (AI) is transforming cybersecurity – but not always for the better. In 2024, cybercriminals are leveraging AI to launch more advanced and targeted attacks. From AI-generated phishing emails to automated hacking tools, the need for AI-driven defences has never been greater. […]